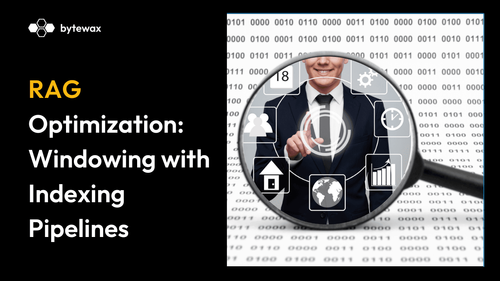
Bytewax is a popular choice to process and embedd real-time data streams from various data sources to any of the leading vector databases such as Qdrant, Pinecone, Elastic, Milvus, Feast, and many more.
Real-time data is now essential for any large language model. Many developers have discovered Bytewax, as a Python-native stream processor, as their go to solution to build real-time feature pipelines and generating embeddings, among other applications.
Bytewax has become an essential tool in the developer community to create real-time LLMs.
Ingest and process continuous data streams from multiple sources, and generate real-time data embeddings. These embeddings update a vector database continuously, supporting GenAI models in tasks such as text generation, image synthesis, or code generation.
Process real-time data streams to dynamically create tailored prompts for GenAI models. This allows for the real-time generation of personalized content, images, or other outputs, ensuring relevance to the current context and user needs.
Ingest and process multiple real-time data streams of different modalities (text, images, audio, video, etc.), fusing them together to create rich, multi-modal inputs for GenAI models, enabling more context-aware and comprehensive generation tasks.
Bytewax is a popular choice to process and embedd real-time data streams from various data sources to any of the leading vector databases such as Qdrant, Pinecone, Elastic, Milvus, Feast, and many more.